Solving Tracking Latency
- teejaydub
- Aug 31, 2016
- 2 min read
During the proof of concept testing, one issue that we ran into was an apparent lag of the tracked vehicle. This was noticeable, but under one second. Yesterday was spent attempting to find the cause of the majority of the latency.
We debugged the Python code, and timed different, individual lines of code to see how long it was taking to run. It was soon discovered that the line that was taking the longest, by a longshot, was the line that was actually grabbing the frame from the webcam. We are using OpenCV with Python to do our minimal image processing and grabbing of the frames. This actually made sense by realizing that the camera framerate was currently at 30fps, and the code was simply waiting for the next frame to arrive. One solution that came to mind immediately was doubling the framerate if possible.
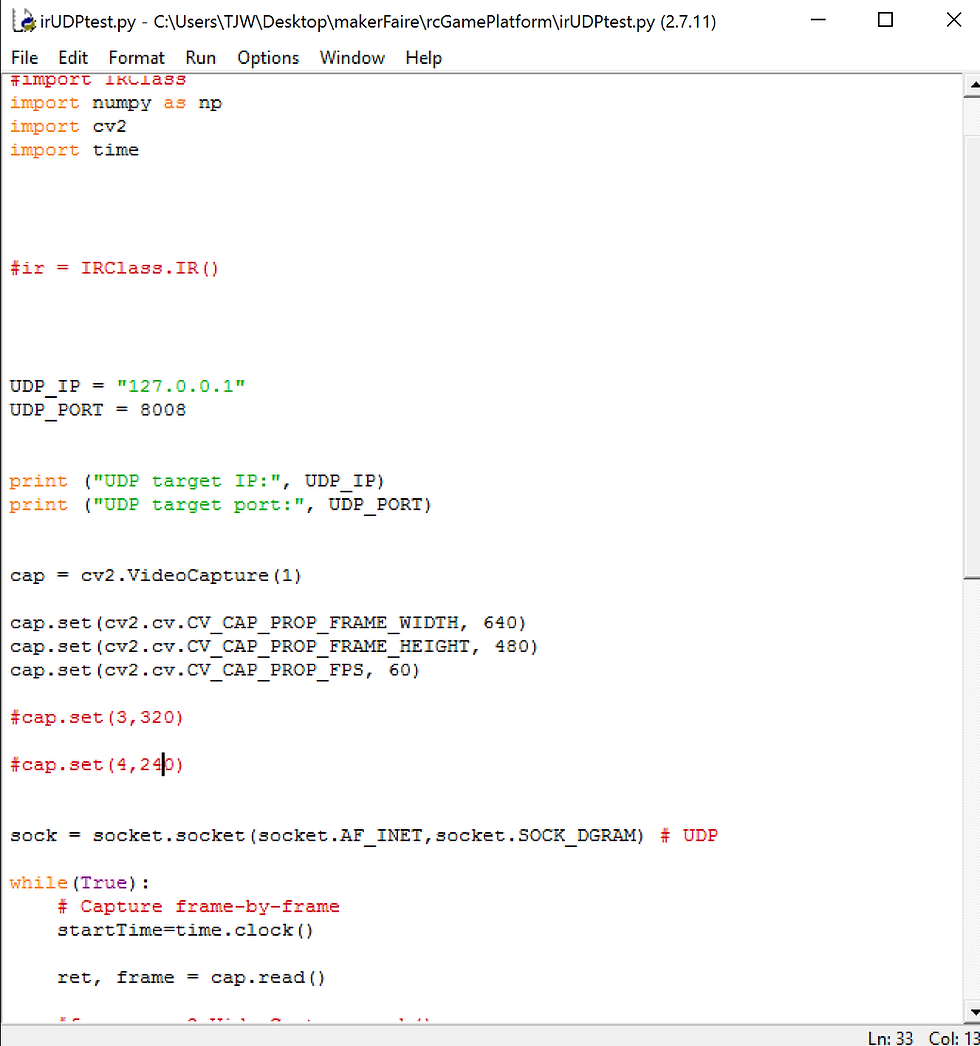
There were initial thoughts that the image processing was taking the longest time. This was the line of code that searched the entire image for the brightest pixel, signifying that there was a car at that location. But, somewhat surprisingly, that line of code was negligible in the amount of time it took to complete. One lesson learned from all of this debugging was that you should profile your code first before assuming what is taking the longest amount of time, because I wasted a bit of time researching how to do GPU accelerated image processing with OpenCV (although undoubtedly useful for something else) when in reality that image processing line wasn't taking up any meaningful time.
We also thought that another point of latency could be arising from the transmission of data from Python to Unity over UDP. The method we came up with to test the transmission latency was by asking Python how many milliseconds its been since Epoch time, then sending it to Unity, where Unity would immediately be asked how many milliseconds its been since Epoch time aka the same question. The values were subtracted and we received the net time it took for the message to be transmitted. Not necessarily the most accurate method but it could at least give us a rough idea, and that idea it gave us was that there was no measurable latency, up to over three decimal places. Which makes sense, since the data isn't really being sent anywhere (the host IP was 127.0.0.1).
The conclusion we came to was that there were two points of latency: The time it takes for Python to grab an image from the webcam, and the inherent input lag of the display (the projector). The former can be solved by increasing the framerate of the camera itself. The latter doesn't really have any solution besides the possibility of finding a value in the settings in the projector to put it in a lower latency "game mode" and turning off all of the automatic image processing functionality such as "auto sharpening" and other features that add latency.
Thanks to Angel for helping out with debugging!
Comments